As the amount of data companies store in the cloud continues to grow and organizations look for new ways to store all of this information, many are turning to data lakes and data warehouses.
As their names suggest, both data lakes and data warehouses are designed for pooling or storing large amounts of data. The main distinction between the two is that while a data lake is a vast pool of raw data, the purpose for which is not yet defined, a data warehouse is a repository for structured, filtered data that has already been processed for a specific purpose.
In both cases, data lakes and data warehouses provide a copy of your data from various source systems, like Salesforce for example. For this reason, it can be tempting to treat these systems as a backup solution for your production data. When you take a closer look however, both data warehouses and data lakes have significant limitations as a backup and recovery solution.
In this blog, we’ll explain why a data lake or data warehouse isn’t a viable backup solution, particularly for Salesforce, and what a true backup solution should look like.
A data lake and data warehouse’s issues as a backup solution
Difficult to see daily backup snapshots
Ideally, to minimize your recovery point objective, you should be backing up your data at least once a day. The ability to back up on-demand is also extremely valuable when you’re making large-scale changes. This way, you can back up your data immediately before making changes, and can easily revert your data in case anything goes wrong.
Since a data warehouse or data lake only has a single copy of the data, it makes it difficult to save points in time and take a snapshot of your data for a given day. So, while you’ll have a view of your current data, what about a view of the way it was yesterday, last month or any other point in time?
No proactive notification or compare utilities
A typical Salesforce environment has integrations, batch updates, cleanups, and code deployments that run regularly, making it difficult to spot data abnormalities yourself. And if it’s malicious activity that’s designed to be hard to detect, you may not be able to spot it at all.
With a data lake or data warehouse, you can't easily compare what data has been lost or modified and what hasn’t, and have no way of being alerted to data inconsistencies. Lots of data is updated throughout the day, so it's important to store the previous values as a daily baseline to identify additions, changes and deletions for both monitoring and alerting purposes.
Compliance Risks
For publicly traded companies, relying on a data lake or data warehouse as a backup solution poses significant compliance risks. Regulations like SOX require reliable, auditable systems to ensure data integrity and recovery, but data lakes and warehouses lack key features like immutable backups, point-in-time recovery, and automated retention policies. These gaps can lead to regulatory fines, reputational damage, and failed audits, making a dedicated backup solution critical for meeting compliance standards.
Manual data retention
One of the reasons data lakes (and to an extent, data warehouses) are so popular is the sheer amount of data that they can store. The downside of this is that it's easy to just keep pouring data into them without a second thought. In this case, it can be easy to lose track of which data actually needs to be there.
Certain industries have specific regulations (like FERPA for education or HIPAA for healthcare) that dictate designated retention periods. Deleting data ahead of schedule can put your organization at risk of non-compliance, resulting in costly fines and other repercussions. On the other end of the spectrum, there are regulations like GDPR that impose a maximum retention period and other data removal requirements.
With a data lake or data warehouse, you’d have to manually set up all of these policies, which can be difficult and put you at risk.
Data export scripts must be consistently updated to support scheme and API changes
Databases like Salesforce are constantly changing (new objects, new fields, etc). To ensure that these values are reflected in your data lake or data warehouse, you would have to manually configure these updates in the sync process. In other words, there is not an automatic update of the mappings.
Data corruptions will be replicated to the “backup”
To get data into your data lake or data warehouse you will first need to extract the data from the source (Salesforce) through SQL or some API, and then load it into the lake or warehouse. When doing this, you can either do a complete extraction of all data available, or you can do an incremental extraction every time you run a sync.
In either case, any data additions, changes and deletions will be replicated out to the data lake or warehouse, causing any undetected data corruptions in Salesforce to be automatically replicated into your data lake or warehouse.
No restore capabilities to move data from the data lake back to Salesforce
While all of the challenges above are significant, the biggest limitation of using a data lake or data warehouse as a backup solution is the inability to restore data from the data lake. When integrating a data lake or data warehouse with Salesforce, it’s a one-way sync from Salesforce to the data lake or data warehouse– you can’t move data back into Salesforce. So, if there is a data loss or corruption in Salesforce, you don’t have the ability to restore “correct” data from your data lake or warehouse back into Salesforce, or be able to keep child data and look-up relationships intact.
What a true Salesforce backup solution looks like
Own Recover enables automated backup and rapid recovery of Salesforce data and metadata with no storage limits. We provide full daily backup snapshots so we are capturing changes daily, allowing for easy comparison between any two points in time. With tools to identify survey changes to an object, filter just unwanted data changes, and restore at the field level, we help customers quickly and easily restore accurate data.
To learn more about what to consider when choosing a Salesforce backup solution, check out our guide, The Buyer’s Guide to Backup and Recovery.
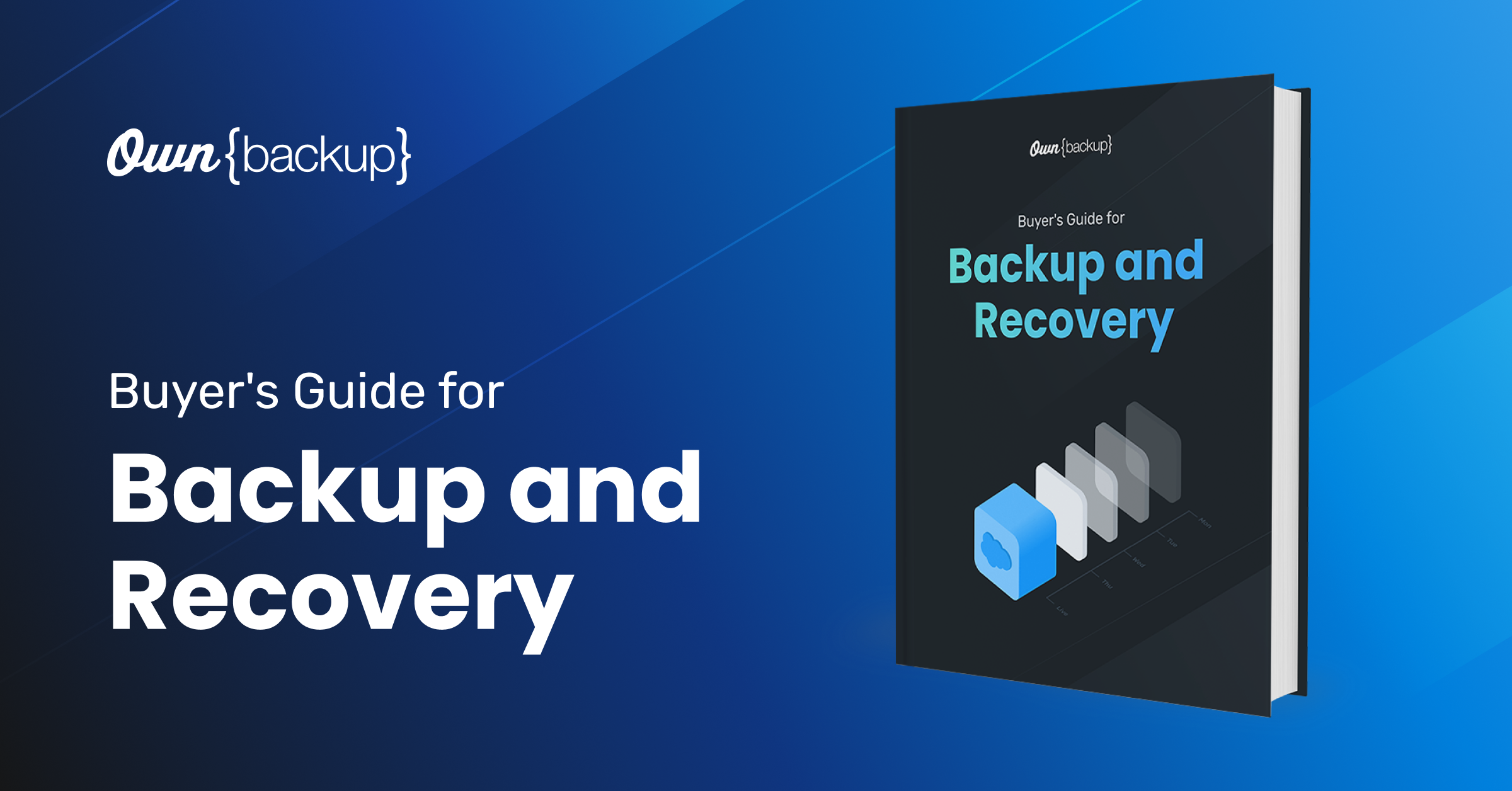